I am a PhD student at UC Berkeley
advised by Prof. Masayoshi Tomizuka
and Prof. Andrea Bajcsy at Carnegie Mellon
University.
My research lies in the intersection of robotics and AI with a focus on
safe alignment between embodied agents and humans.
I tackle the alignment and safety problems that emerge throughout the
life-cycle of foundation models in robotics, ranging from: training (wherein we need to collect
and quantify what kinds of embodied data will enable the desired robotics capabilities), to
fine-tuning (wherein we must align these models with humans), to deployment (where these models
must run in real-time, reliably detect out-of-distribution scenarios, and confidently hand over
control to fallback-strategies).
I ground my work through a variety of applications, from autonomous cars, to personalized robots, to
generative AI and in experiments with real human participants.
During my PhD study, I also spent a significant amount of time at Waymo, scaling my research outcomes in driving foundation
models, including pre-training, post-training preference alignment, and distillation for onboard
deployment.
I am fortunate to have the opportunity to work at NVIDIA Research, focusing on
vision-language-action models for autonomous driving.
Previously, I was a research intern at WeRide,
Honda Research Institute, and Qualcomm AI
Research.
google
scholar   |  
X   
|
|
Representative Publications
For the most up-to-date list of publications, please see google scholar.
|
🎯 Post-training Alignment
|
|
Direct Post-Training Preference Alignment for Multi-Agent Motion Generation Model Using
Implicit Feedback from Pre-training Demonstrations
Ran Tian, Kratarth Goel
International Conference on Learning Representations (ICLR), 2025,
Spotlight paper
paper
 
website
|
|
Maximizing Alignment with Minimal Feedback: Efficiently Learning Rewards for Visuomotor
Robot Policy Alignment
Ran Tian, Yilin Wu, Chenfeng Xu, Masayoshi Tomizuka, Jitendra Malik, Andrea Bajcsy
arXiv, 2024
paper
 
website
|
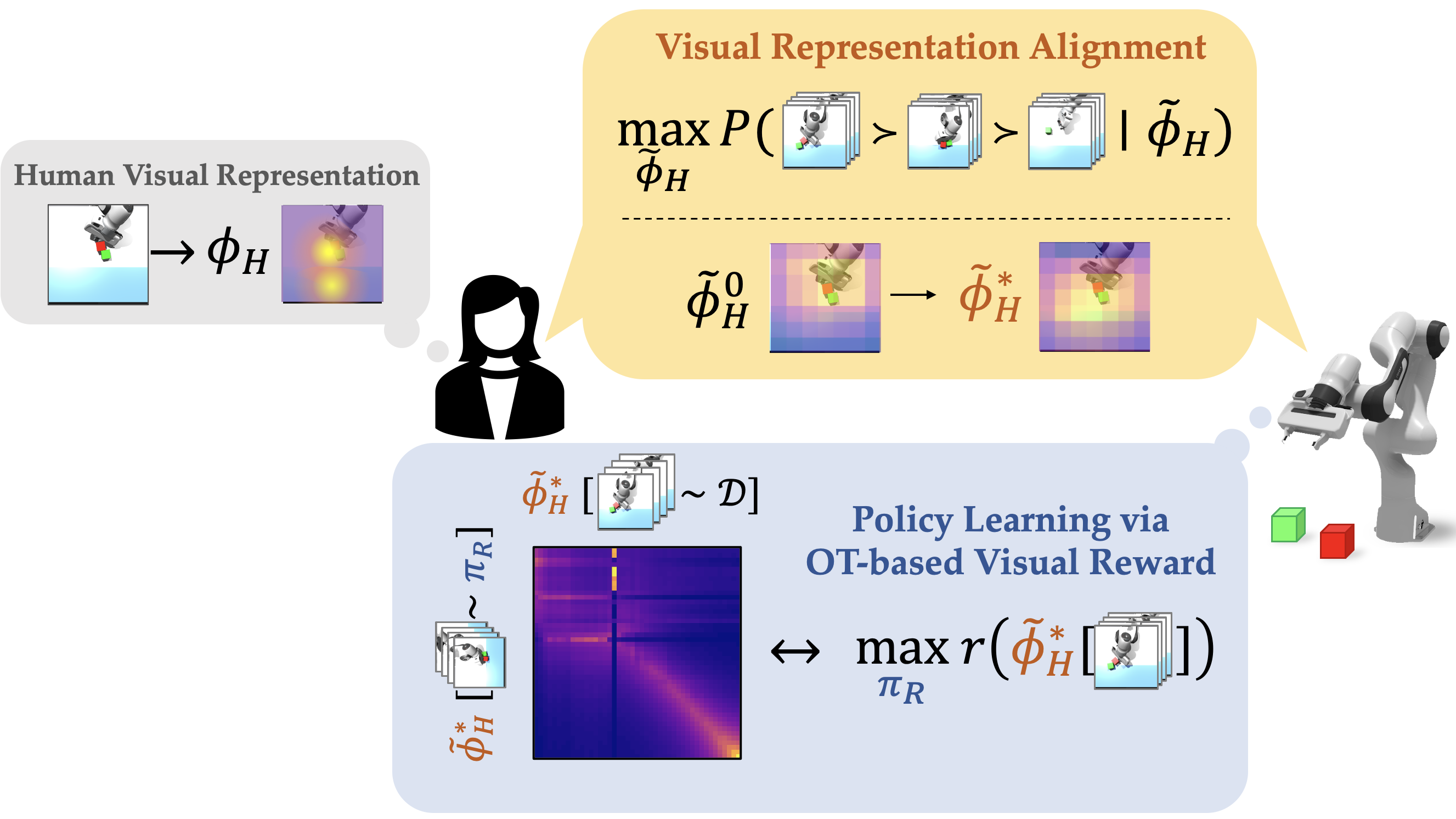 |
What Matters to You? Towards Visual Representation Alignment for Robot Learning
Ran Tian, Chenfeng Xu, Masayoshi Tomizuka, Jitendra Malik, Andrea Bajcsy
International Conference on Learning Representations (ICLR), 2024
paper
 
|
⚙️ Onboard Deployment Efficiency and Safety
|
|
From Foresight to Forethought: VLM-In-the-Loop Policy Steering via Latent Alignment
Yilin Wu, Ran Tian, Gouku Swamy, Andrea Bajcsy
arXiv, 2025
ICLR Workshop on World Models, 2025,
Oral paper.
paper
 
website
|
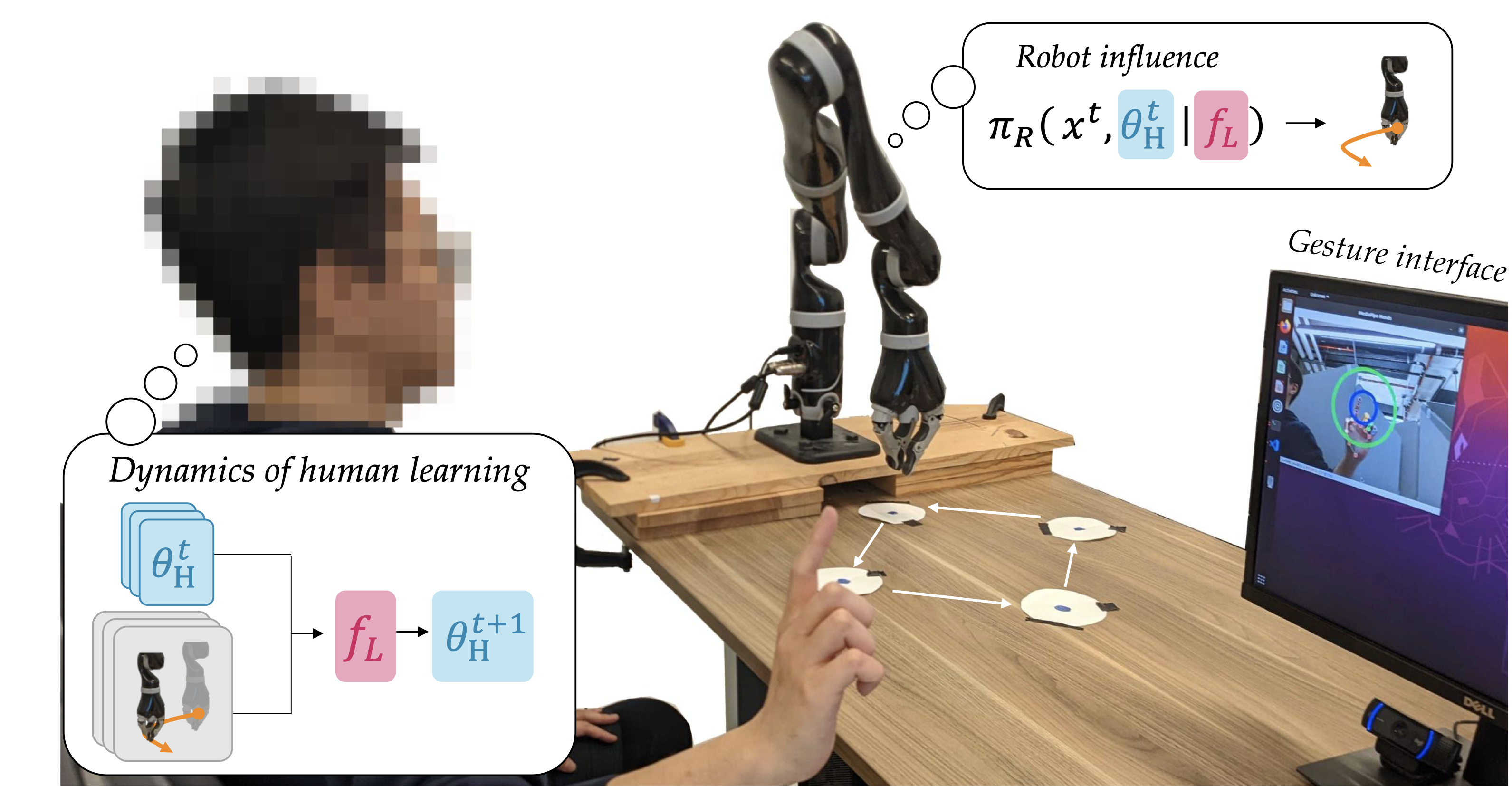 |
Towards Modeling and Influencing the Dynamics of Human Learning
Ran Tian, Masayoshi Tomizuka, Anca Dragan, Andrea Bajcsy
International Conference on Human-Robot Interaction (HRI), 2023
paper
 
talk
|
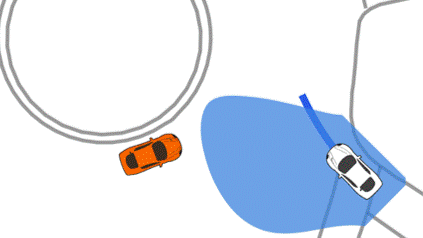 |
Safety Assurances for Human-Robot Interaction via Confidence-aware Game-theoretic Human Models
Ran Tian, Liting Sun, Andrea Bajcsy, Masayoshi Tomizuka, Anca Dragan
International Conference on Robotics and Automation (ICRA), 2022
paper
 
talk
|
🧩 Representation and Policy Pre-training
|
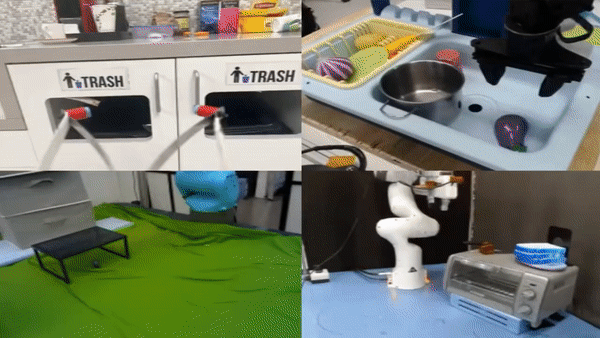 |
Open X-Embodiment: Robotic Learning Datasets and RT-X Models
Google, Ran Tian, et al.
International Conference on Robotics and Automation (ICRA), 2024,
Best paper, best student paper, and best manipulation paper
paper
 
website
|
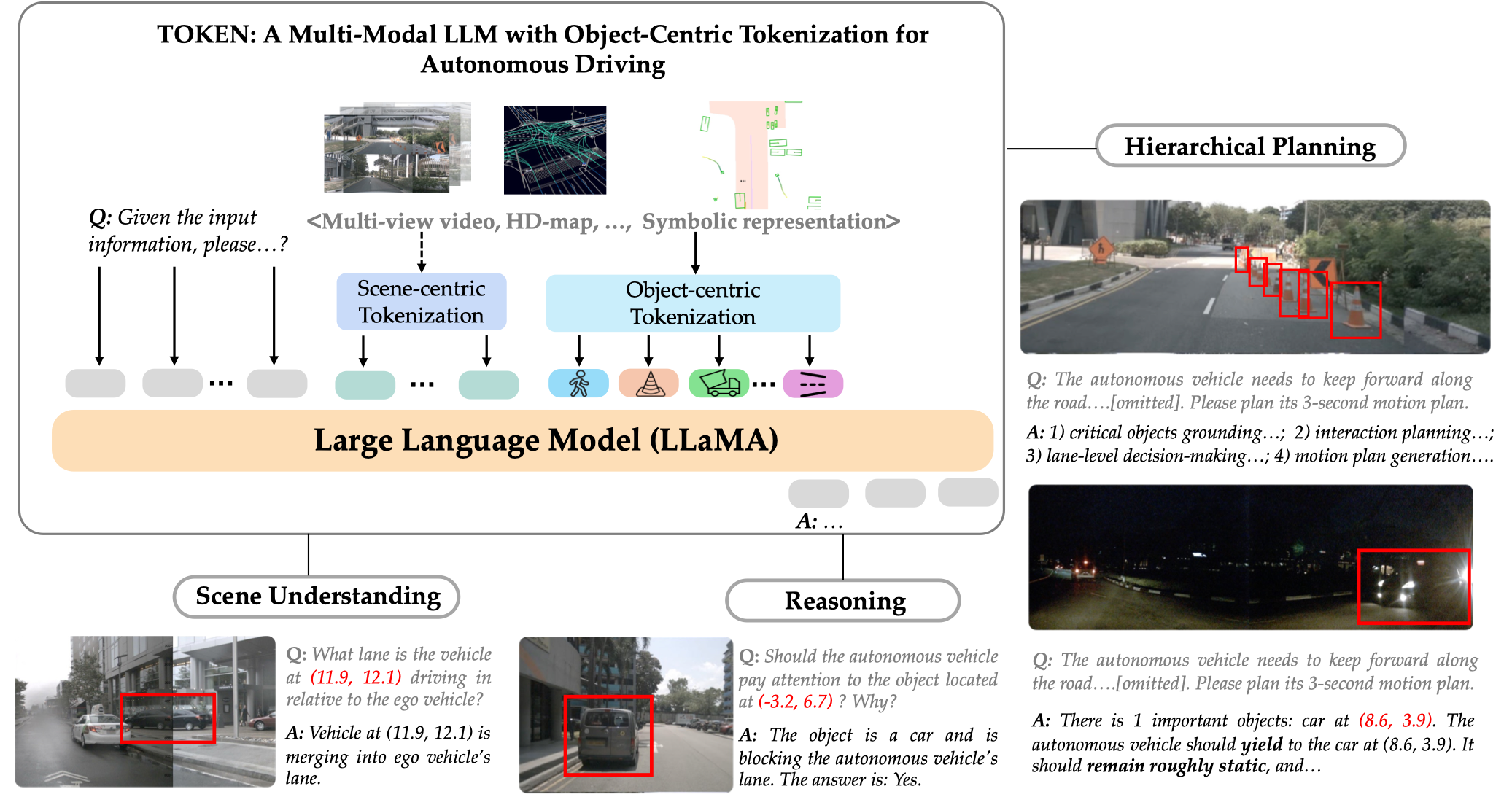 |
Tokenize the World into Object-level Knowledge to Address Long-tail Events in Autonomous
Driving
Ran Tian, Boyi Li, Xinshuo Weng, Yuxiao Chen, Edward Schmerling, Yue Wang, Boris Ivanovic, Marco
Pavone
Conference on Robot Learning (CoRL), 2024
paper
 
website
|
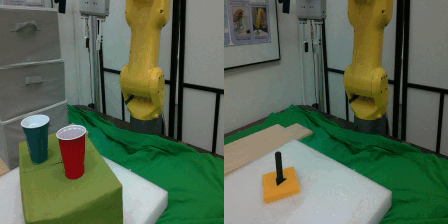 |
Human-oriented Representation Learning for Robotic Manipulation
Mingxiao Huo, Mingyu Ding, Chenfeng Xu, Ran Tian, Xinghao Zhu, Yao Mu Lingfeng Sun, Masayoshi
Tomizuka,
Wei Zhan
Robotics: Science and Systems, 2024
paper
 
website
|
|